Publications
Combined Object Categorization and Segmentation with an Implicit Shape Model
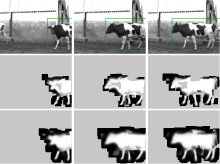
We present a method for object categorization in real-world scenes. Following a common consensus in the field, we do not assume that a figureground segmentation is available prior to recognition. However, in contrast to most standard approaches for object class recognition, our approach automatically segments the object as a result of the categorization. This combination of recognition and segmentation into one process is made possible by our use of an Implicit Shape Model, which integrates both into a common probabilistic framework. In addition to the recognition and segmentation result, it also generates a per-pixel confidence measure specifying the area that supports a hypothesis and how much it can be trusted. We use this confidence to derive a natural extension of the approach to handle multiple objects in a scene and resolve ambiguities between overlapping hypotheses with a novel MDL-based criterion. In addition, we present an extensive evaluation of our method on a standard dataset for car detection and compare its performance to existing methods from the literature. Our results show that the proposed method significantly outperforms previously published methods while needing one order of magnitude less training examples. Finally, we present results for articulated objects, which show that the proposed method can categorize and segment unfamiliar objects in different articulations and with widely varying texture patterns, even under significant partial occlusion.
Scale Invariant Object Categorization Using a Scale-Adaptive Mean-Shift Search
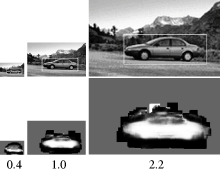
The goal of our work is object categorization in real-world scenes. That is, given a novel image we want to recognize and localize unseen-before objects based on their similarity to a learned object category. For use in a realworld system, it is important that this includes the ability to recognize objects at multiple scales. In this paper, we present an approach to multi-scale object categorization using scale-invariant interest points and a scale-adaptive Mean-Shift search. The approach builds on the method from [12], which has been demonstrated to achieve excellent results for the single-scale case, and extends it to multiple scales. We present an experimental comparison of the influence of different interest point operators and quantitatively show the method’s robustness to large scale changes.
Awarded the main prize of the German Pattern Recognition Society (DAGM Best Paper Award)
Interleaved Object Categorization and Segmentation
This thesis is concerned with the problem of visual object categorization, that is of recognizing unseen-before objects, localizing them in cluttered real-world images, and assigning the correct category label. This capability is one of the core competencies of the human visual system. Yet, computer vision systems are still far from reaching a comparable level of performance. Moreover, computer vision research has in the past mainly focused on the simpler and more specific problem of identifying known objects under novel viewing conditions. The visual categorization problem is closely linked to the task of figure-ground segmentation, that is of dividing the image into an object and a non-object part. Historically, figure-ground segmentation has often been seen as an important and even necessary preprocessing step for object recognition. However, purely bottomup approaches have so far been unable to yield segmentations of sufficient quality, so that most current recognition approaches have been designed to work independently from segmentation. In contrast, this thesis considers object categorization and figure-ground segmentation as two interleaved processes that closely collaborate towards a common goal. The core part of our work is a probabilistic formulation which integrates both capabilities into a common framework. As shown in our experiments, the tight coupling between those two processes allows them to profit from each other and improve their individual performances. The resulting approach can detect categorical objects in novel images and automatically compute a segmentation for them. This segmentation is then used to again improve recognition by allowing the system to focus its effort on object pixels and discard misleading influences from the background. In addition to improving the recognition performance for individual hypotheses, the top-down segmentation also allows to determine exactly from where a hypothesis draws its support. We use this information to design a hypothesis verification stage based on the MDL principle that resolves ambiguities between overlapping hypotheses on a per-pixel level and factors out the effects of partial occlusion. Altogether, this procedure constitutes a novel mechanism in object detection that allows to analyze scenes containing multiple objects in a principled manner. Our results show that it presents an improvement over conventional criteria based on bounding box overlap and permits more accurate acceptance decisions. Our approach is based on a highly flexible implicit representation for object shape that can combine the information of local parts observed on different training examples and interpolate between the corresponding objects. As a result, the proposed method can learn object models already from few training examples and achieve competitive object detection performance with training sets that are between one and two orders of magnitude smaller than those used in comparable systems. An extensive evaluation on several large data sets shows that the system is applicable to many different object categories, including both rigid and articulated objects.
Previous Year (2003)