Profile
![]() |
Publications
Global Hierarchical Attention for 3D Point Cloud Analysis
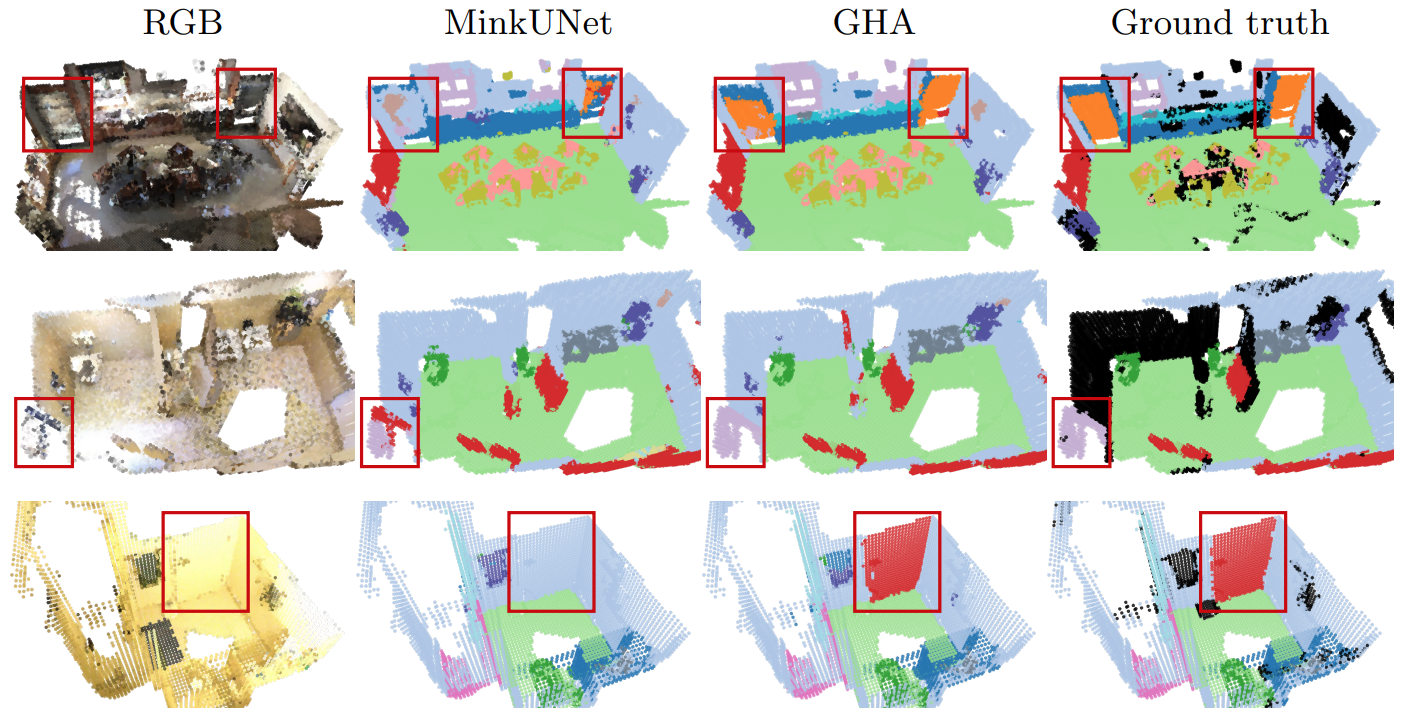
We propose a new attention mechanism, called Global Hierarchical Attention (GHA), for 3D point cloud analysis. GHA approximates the regular global dot-product attention via a series of coarsening and interpolation operations over multiple hierarchy levels. The advantage of GHA is two-fold. First, it has linear complexity with respect to the number of points, enabling the processing of large point clouds. Second, GHA inherently possesses the inductive bias to focus on spatially close points, while retaining the global connectivity among all points. Combined with a feedforward network, GHA can be inserted into many existing network architectures. We experiment with multiple baseline networks and show that adding GHA consistently improves performance across different tasks and datasets. For the task of semantic segmentation, GHA gives a +1.7% mIoU increase to the MinkowskiEngine baseline on ScanNet. For the 3D object detection task, GHA improves the CenterPoint baseline by +0.5% mAP on the nuScenes dataset, and the 3DETR baseline by +2.1% mAP25 and +1.5% mAP50 on ScanNet.
Pedestrian-Robot Interactions on Autonomous Crowd Navigation: Reactive Control Methods and Evaluation Metrics

Autonomous navigation in highly populated areas remains a challenging task for robots because of the difficulty in guaranteeing safe interactions with pedestrians in unstructured situations. In this work, we present a crowd navigation control framework that delivers continuous obstacle avoidance and post-contact control evaluated on an autonomous personal mobility vehicle. We propose evaluation metrics for accounting efficiency, controller response and crowd interactions in natural crowds. We report the results of over 110 trials in different crowd types: sparse, flows, and mixed traffic, with low- (< 0.15 ppsm), mid- (< 0.65 ppsm), and high- (< 1 ppsm) pedestrian densities. We present comparative results between two low-level obstacle avoidance methods and a baseline of shared control. Results show a 10% drop in relative time to goal on the highest density tests, and no other efficiency metric decrease. Moreover, autonomous navigation showed to be comparable to shared-control navigation with a lower relative jerk and significantly higher fluency in commands indicating high compatibility with the crowd. We conclude that the reactive controller fulfills a necessary task of fast and continuous adaptation to crowd navigation, and it should be coupled with high-level planners for environmental and situational awareness.
2D vs. 3D LiDAR-based Person Detection on Mobile Robots
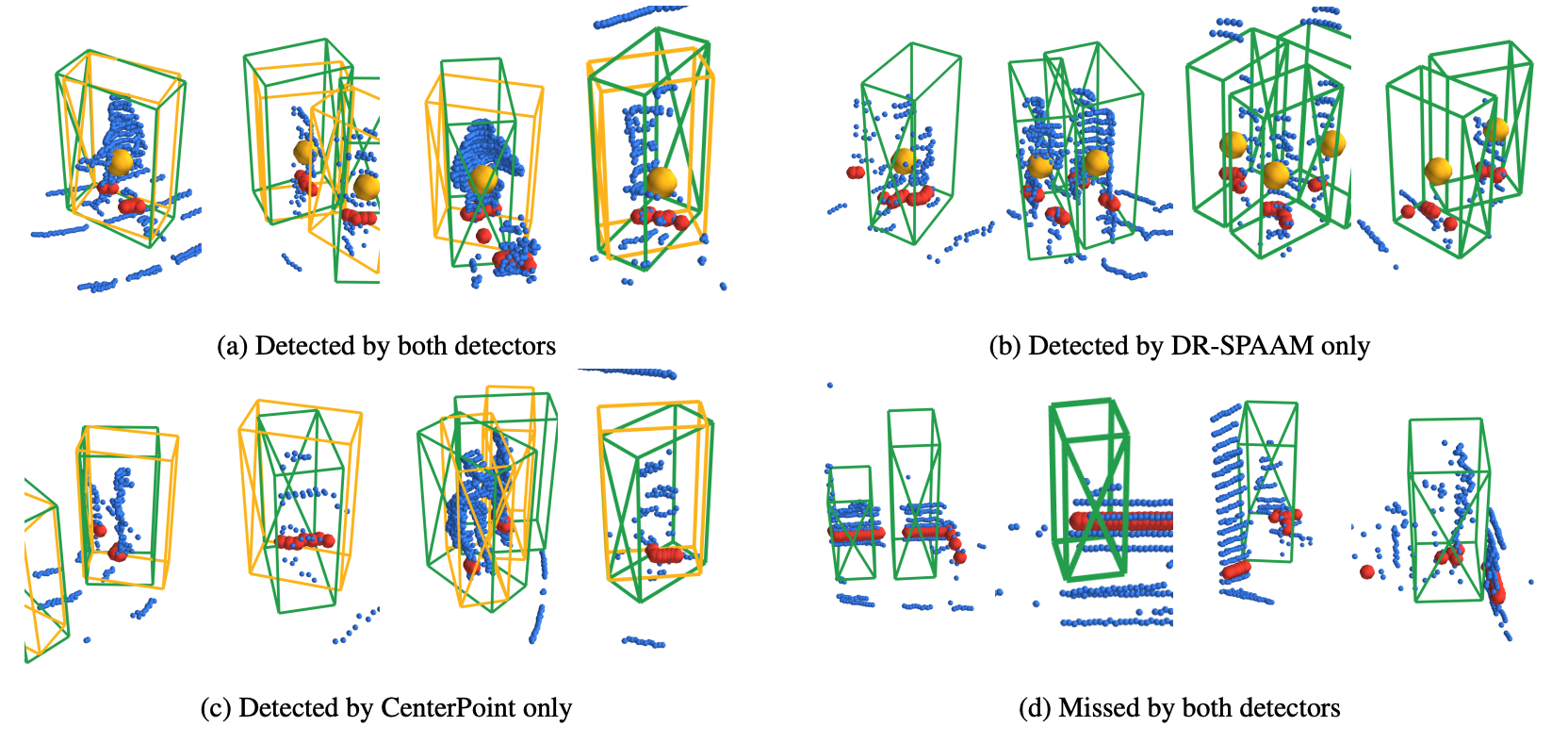
Person detection is a crucial task for mobile robots navigating in human-populated environments. LiDAR sensors are promising for this task, thanks to their accurate depth measurements and large field of view. Two types of LiDAR sensors exist: the 2D LiDAR sensors, which scan a single plane, and the 3D LiDAR sensors, which scan multiple planes, thus forming a volume. How do they compare for the task of person detection? To answer this, we conduct a series of experiments, using the public, large-scale JackRabbot dataset and the state-of-the-art 2D and 3D LiDAR-based person detectors (DR-SPAAM and CenterPoint respectively). Our experiments include multiple aspects, ranging from the basic performance and speed comparison, to more detailed analysis on localization accuracy and robustness against distance and scene clutter. The insights from these experiments highlight the strengths and weaknesses of 2D and 3D LiDAR sensors as sources for person detection, and are especially valuable for designing mobile robots that will operate in close proximity to surrounding humans (e.g. service or social robot).
Person-MinkUNet: 3D Person Detection with LiDAR Point Cloud
In this preliminary work we attempt to apply submanifold sparse convolution to the task of 3D person detection. In particular, we present Person-MinkUNet, a single-stage 3D person detection network based on Minkowski Engine with U-Net architecture. The network achieves a 76.4% average precision (AP) on the JRDB 3D detection benchmark.
Winner of JRDB 3D detection challenge in JRDB-ACT Workshop at CVPR 2021
Self-Supervised Person Detection in 2D Range Data using a Calibrated Camera
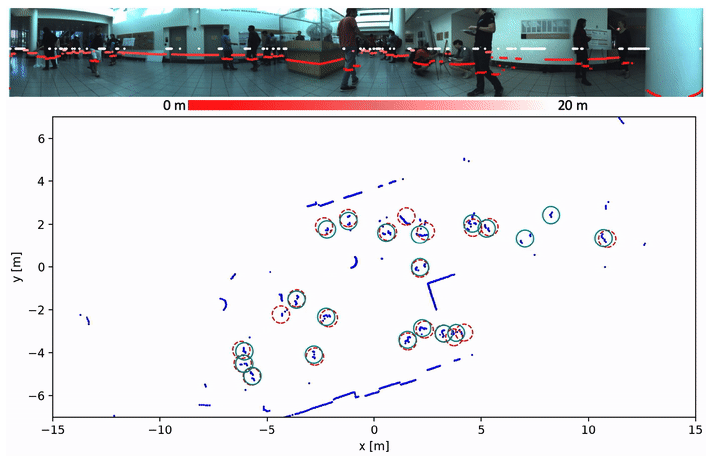
Deep learning is the essential building block of state-of-the-art person detectors in 2D range data. However, only a few annotated datasets are available for training and testing these deep networks, potentially limiting their performance when deployed in new environments or with different LiDAR models. We propose a method, which uses bounding boxes from an image-based detector (e.g. Faster R-CNN) on a calibrated camera to automatically generate training labels (called pseudo-labels) for 2D LiDAR-based person detectors. Through experiments on the JackRabbot dataset with two detector models, DROW3 and DR-SPAAM, we show that self- supervised detectors, trained or fine-tuned with pseudo-labels, outperform detectors trained using manual annotations from a different dataset. Combined with robust training techniques, the self-supervised detectors reach a performance close to the ones trained using manual annotations. Our method is an effective way to improve person detectors during deployment without any additional labeling effort, and we release our source code to support relevant robotic applications.
DR-SPAAM: A Spatial-Attention and Auto-regressive Model for Person Detection in 2D Range Data

Detecting persons using a 2D LiDAR is a challenging task due to the low information content of 2D range data. To alleviate the problem caused by the sparsity of the LiDAR points, current state-of-the-art methods fuse multiple previous scans and perform detection using the combined scans. The downside of such a backward looking fusion is that all the scans need to be aligned explicitly, and the necessary alignment operation makes the whole pipeline more expensive -- often too expensive for real-world applications. In this paper, we propose a person detection network which uses an alternative strategy to combine scans obtained at different times. Our method, Distance Robust SPatial Attention and Auto-regressive Model (DR-SPAAM), follows a forward looking paradigm. It keeps the intermediate features from the backbone network as a template and recurrently updates the template when a new scan becomes available. The updated feature template is in turn used for detecting persons currently in the scene. On the DROW dataset, our method outperforms the existing state-of-the-art, while being approximately four times faster, running at 87.2 FPS on a laptop with a dedicated GPU and at 22.6 FPS on an NVIDIA Jetson AGX embedded GPU. We release our code in PyTorch and a ROS node including pre-trained models.
Jetson project of the month for September 2020