Exploring Spatial Context for 3D Semantic Segmentation of Point Clouds
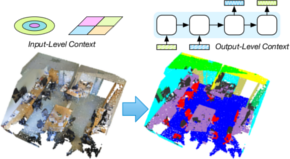
Deep learning approaches have made tremendous progress in the field of semantic segmentation over the past few years. However, most current approaches operate in the 2D image space. Direct semantic segmentation of unstructured 3D point clouds is still an open research problem. The recently proposed PointNet architecture presents an interesting step ahead in that it can operate on unstructured point clouds, achieving decent segmentation results. However, it subdivides the input points into a grid of blocks and processes each such block individually. In this paper, we investigate the question how such an architecture can be extended to incorporate larger-scale spatial context. We build upon PointNet and propose two extensions that enlarge the receptive field over the 3D scene. We evaluate the proposed strategies on challenging indoor and outdoor datasets and show improved results in both scenarios.
Video
Downloads
Contact
- engelmann@vision.rwth-aachen.de
- kontogianni@vision.rwth-aachen.de
Datasets
We evaluated our method on the following datasets:
- Stanford Large-Scale 3D Indoor Spaces Dataset (S3DIS) - Link
- Virtual KITTI 3D Semantic Segmentation (VKITTI3D) - Link
Citation
@inproceedings{3dsemseg_ICCVW17, author = {Francis Engelmann and Theodora Kontogianni and Alexander Hermans and Bastian Leibe}, title = {Exploring Spatial Context for 3D Semantic Segmentation of Point Clouds}, booktitle = {{IEEE} International Conference on Computer Vision, 3DRMS Workshop, {ICCV}}, year = {2017} }