Welcome

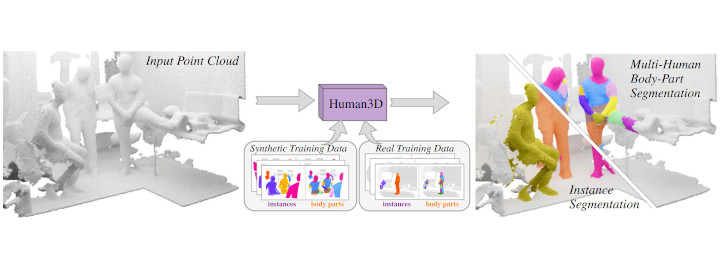
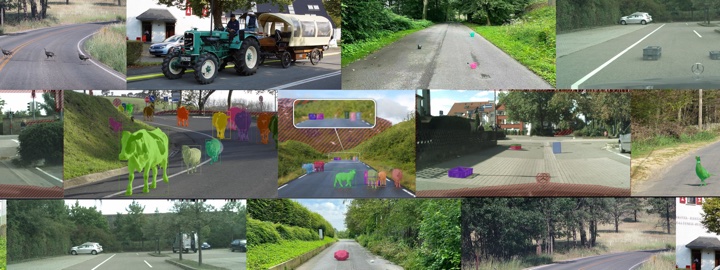
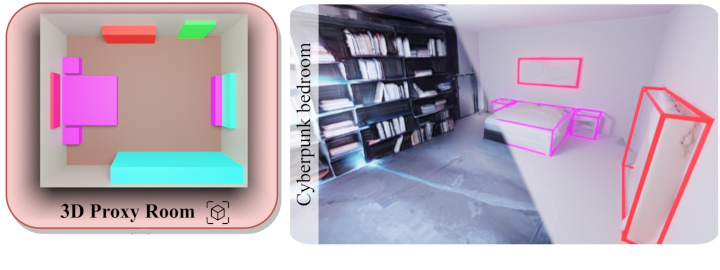
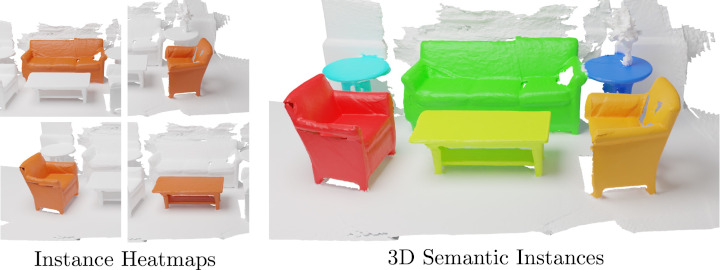
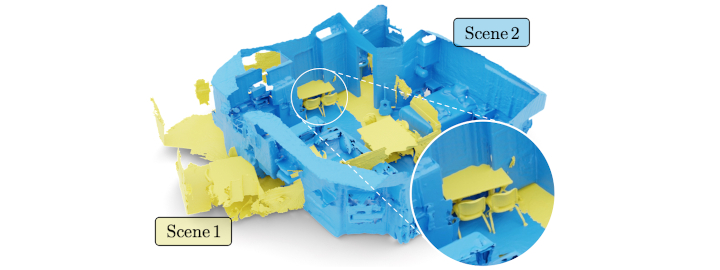
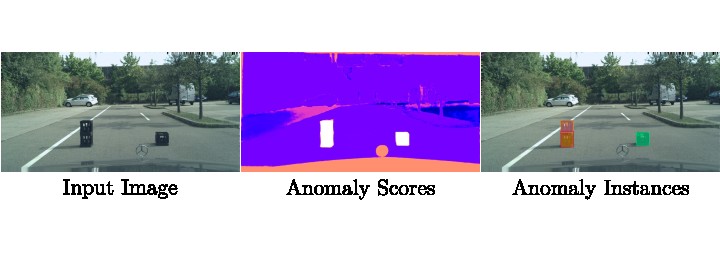
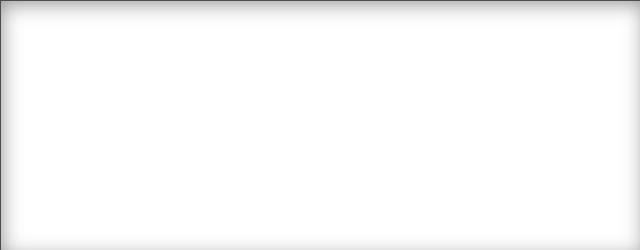
Welcome to the Computer Vision Group at RWTH Aachen University!
The Computer Vision group has been established at RWTH Aachen University in context with the Cluster of Excellence "UMIC - Ultra High-Speed Mobile Information and Communication" and is associated with the Chair Computer Sciences 8 - Computer Graphics, Computer Vision, and Multimedia. The group focuses on computer vision applications for mobile devices and robotic or automotive platforms. Our main research areas are visual object recognition, tracking, self-localization, 3D reconstruction, and in particular combinations between those topics.
We offer lectures and seminars about computer vision and machine learning.
You can browse through all our publications and the projects we are working on.
Important information for the Wintersemester 2023/2024: Unfortunately the following lectures are not offered in this semester: a) Computer Vision 2 b) Advanced Machine Learning
News
• |
RO-MAN'25 Our paper How do Foundation Models Compare to Skeleton-Based Approaches for Gesture Recognition in Human-Robot Interaction? has been accepted! |
June 12, 2025 |
• |
CVPR'25 We have two papers accepted at Conference on Computer Vision and Pattern Recognition (CVPR) 2025! |
May 5, 2025 |
• |
ICRA'25 We have four papers at the IEEE International Conference on Robotics and Automation (ICRA). See you all in Atlanta! |
Feb. 20, 2025 |
• |
WACV'25 Our work "Fine-Tuning Image-Conditional Diffusion Models is Easier than You Think" has been accepted at WACV'25. |
Nov. 18, 2024 |
• |
IROS'24 Our work "Look Gauss, No Pose: Novel View Synthesis using Gaussian Splatting without Accurate Pose Initialization" has been accepted at IROS'24. |
July 30, 2024 |
• |
CVPR'24 We have two papers accepted at the 2024 IEEE Conference on Computer Vision and Pattern Recognition (CVPR):
We have two papers accepted at Workshops: |
Feb. 27, 2024 |
Recent Publications
![]() DONUT: A Decoder-Only Model for Trajectory Prediction International Conference on Computer Vision (ICCV) 2025 Predicting the motion of other agents in a scene is highly relevant for autonomous driving, as it allows a self-driving car to anticipate. Inspired by the success of decoder-only models for language modeling, we propose DONUT, a Decoder-Only Network for Unrolling Trajectories. Different from existing encoder-decoder forecasting models, we encode historical trajectories and predict future trajectories with a single autoregressive model. This allows the model to make iterative predictions in a consistent manner, and ensures that the model is always provided with up-to-date information, enhancing the performance. Furthermore, inspired by multi-token prediction for language modeling, we introduce an 'overprediction' strategy that gives the network the auxiliary task of predicting trajectories at longer temporal horizons. This allows the model to better anticipate the future, and further improves the performance. With experiments, we demonstrate that our decoder-only approach outperforms the encoder-decoder baseline, and achieves new state-of-the-art results on the Argoverse 2 single-agent motion forecasting benchmark. ![]() |
![]() Fine-Tuning Image-Conditional Diffusion Models is Easier than You Think Winter Conference on Computer Vision (WACV) 2025 Recent work showed that large diffusion models can be reused as highly precise monocular depth estimators by casting depth estimation as an image-conditional image generation task. While the proposed model achieved state-of-the-art results, high computational demands due to multi-step inference limited its use in many scenarios. In this paper, we show that the perceived inefficiency was caused by a flaw in the inference pipeline that has so far gone unnoticed. The fixed model performs comparably to the best previously reported configuration while being more than 200x faster. To optimize for downstream task performance, we perform end-to-end fine-tuning on top of the single-step model with task-specific losses and get a deterministic model that outperforms all other diffusion-based depth and normal estimation models on common zero-shot benchmarks. We surprisingly find that this fine-tuning protocol also works directly on Stable Diffusion and achieves comparable performance to current state-of-the-art diffusion-based depth and normal estimation models, calling into question some of the conclusions drawn from prior works. ![]() |
![]() Interactive4D: Interactive 4D LiDAR Segmentation International Conference on Robotics and Automation (ICRA) 2025 Interactive segmentation has an important role in facilitating the annotation process of future LiDAR datasets. Existing approaches sequentially segment individual objects at each LiDAR scan, repeating the process throughout the entire sequence, which is redundant and ineffective. In this work, we propose interactive 4D segmentation, a new paradigm that allows segmenting multiple objects on multiple LiDAR scans simultaneously, and Interactive4D, the first interactive 4D segmentation model that segments multiple objects on superimposed consecutive LiDAR scans in a single iteration by utilizing the sequential nature of LiDAR data. While performing interactive segmentation, our model leverages the entire space-time volume, leading to more efficient segmentation. Operating on the 4D volume, it directly provides consistent instance IDs over time and also simplifies tracking annotations. Moreover, we show that click simulations are crucial for successful model training on LiDAR point clouds. To this end, we design a click simulation strategy that is better suited for the characteristics of LiDAR data. To demonstrate its accuracy and effectiveness, we evaluate Interactive4D on multiple LiDAR datasets, where Interactive4D achieves a new state-of-the-art by a large margin. ![]() |