Direct Shot Correspondence Matching
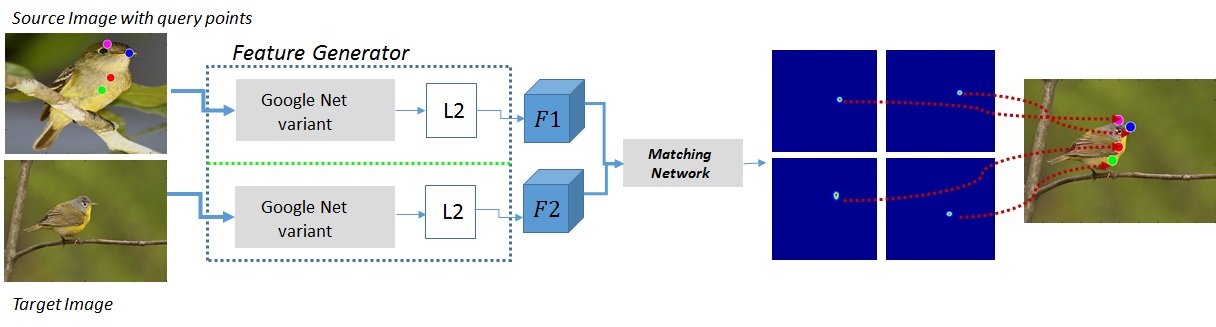
We propose a direct shot method for the task of correspondence matching. Instead of minimizing a loss based on positive and negative pairs, which requires hard-negative mining step for training and nearest neighbor search step for inference, we propose a novel similarity heatmap generator that makes these additional steps obsolete. The similarity heatmap generator efficiently generates peaked similarity heatmaps over the target image for all the query keypoints in a single pass. The matching network can be appended to any standard deep network architecture to make it end-to-end trainable with N-pairs based metric learning and achieves superior performance. We evaluate the proposed method on various correspondence matching datasets and achieve state-of-the-art performance.