Online Adaptation of Convolutional Neural Networks for Video Object Segmentation
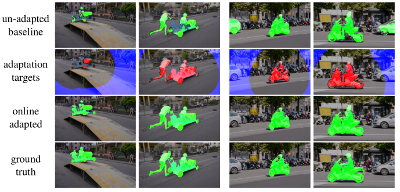
We tackle the task of semi-supervised video object segmentation, i.e. segmenting the pixels belonging to an object in the video using the ground truth pixel mask for the first frame. We build on the recently introduced one-shot video object segmentation (OSVOS) approach which uses a pretrained network and fine-tunes it on the first frame. While achieving impressive performance, at test time OSVOS uses the fine-tuned network in unchanged form and is not able to adapt to large changes in object appearance. To overcome this limitation, we propose Online Adaptive Video Object Segmentation (OnAVOS) which updates the network online using training examples selected based on the confidence of the network and the spatial configuration. Additionally, we add a pretraining step based on objectness, which is learned on PASCAL. Our experiments show that both extensions are highly effective and improve the state of the art on DAVIS to an intersection-over-union score of 85.7%.
@inproceedings{voigtlaender17BMVC,
author = {Paul Voigtlaender and Bastian Leibe},
title = {Online Adaptation of Convolutional Neural Networks for Video Object Segmentation},
booktitle = {BMVC},
year = {2017}
}