PatchIt: Self-supervised Network Weight Initialization for Fine-grained Recognition
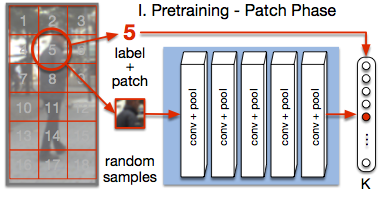
ConvNet training is highly sensitive to initialization of the weights. A widespread approach is to initialize the network with weights trained for a different task, an auxiliary task. The ImageNet-based ILSVRC classification task is a very popular choice for this, as it has shown to produce powerful feature representations applicable to a wide variety of tasks. However, this creates a significant entry barrier to exploring non-standard architectures. In this paper, we propose a self-supervised pretraining, the PatchTask, to obtain weight initializations for fine-grained recognition problems, such as person attribute recognition, pose estimation, or action recognition. Our pretraining allows us to leverage additional unlabeled data from the same source, which is often readily available, such as detection bounding boxes. We experimentally show that our method outperforms a standard random initialization by a considerable margin and closely matches the ImageNet-based initialization.
@InProceedings{Sudowe16BMVC,
author = {Patrick Sudowe and Bastian Leibe},
title = {{PatchIt: Self-Supervised Network Weight Initialization for Fine-grained Recognition}},
booktitle = BMVC,
year = {2016}
}