An Efficient Convolutional Network for Human Pose Estimation
British Machine Vision Conference (BMVC'16)
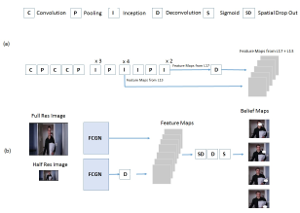
In recent years, human pose estimation has greatly benefited from deep learning and huge gains in performance have been achieved. The trend to maximise the accuracy on benchmarks, however, resulted in computationally expensive deep network architectures that require expensive hardware and pre-training on large datasets. This makes it difficult to compare different methods and to reproduce existing results. We therefore propose in this work an efficient deep network architecture that can be efficiently trained on mid-range GPUs without the need of any pre-training. Despite of the low computational requirements of our network, it is on par with much more complex models on popular benchmarks for human pose estimation.